中文对话大模型ELLE(BE
Large Language Model Engine),基于BLOOM和LLAMA针对中文做了优化,模型调优仅使用由ChatGPT生成的数据,为中文指令提供更好的支持。
目前可知,已经开源了如下内容,日后会陆续更新
1.150万中文指令微调数据集
2.以Bloomz-7b1-mt(70亿参数)为基础,分别在20万,60万,100万,200万数据上进行指令微调后得到的模型Checkpoint。
3.以LLAMA-7b(70亿参数)为基础,分别在60万,200万数据上进行指令微调后得到的模型Checkpoint。
4.对以上模型进行量化后的轻量化模型,便于部署、推理。
我们这次介绍的是在centos 7.9环境下的的部署实践!
1、
下载模型文件
1)
安装git-lfs(默认git已安装)
yum
install git-lfs
2)
初始化git-lfs
/usr/bin/git-lfs
install
3)
下载模型bloomz-7b1-mt
git
clone https://huggingface.co/bigscience/bloomz-7b1-mt
若出现错误:

参考 :
https://www.cnblogs.com/qiumingcheng/p/12402719.html
git-lfs
install --skip-smudge #跳过smudge
git
clone #克隆github文件,大文件为LFS的一个地址
进入项目目录git-lfs pull #将大文件pull回来
git-lfs
install --force #恢复smudge
2、
下载belle项目
Git
clone https://github.com/LianjiaTech/BELLE.git
3、
安装虚拟环境(conda)
conda
create --name env_belle python=3.8
4、
安装依赖包
进入BELLE/train
conda
activate env_belle
pip
install -r requirements.txt -i https://pypi.tuna.tsinghua.edu.cn/simple
5、
启动WebUI
python
interface.py --model_name_or_path /xxxx/bloomz-7b1-mt --ckpt_path /xxxx/bloomz-7b1-mt
Cpu:内存约占用27G
Gpu:int8模式下,显存约占用9G左右
注:直接使用原始模型,则model_name_or_path 和ckpt_path均指定原始模型路径即可
gpu:
6、
模型使用
http://ip:17860/
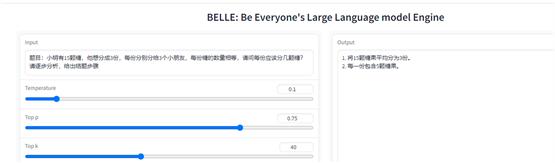