时间过得真快,写上一篇文到现在已经过去半个月之多!LangChain 和 RAG 领域不出意外的又出了不少新思路:
时间过得真快,写上一篇文到现在已经过去半个月之多!LangChain 和 RAG 领域不出意外的又出了不少新思路:
1.
比如 RA-DIT 试图通过增强 RAG 用到的 LLM + 单独训练一个 retriever 的方式加强 LLM 「自有」知识和外挂知识的双向奔赴,效果有些提升但说实话好像不是很稳定。
2.
为了在「外挂知识」这边增强 retrieval
效果,LangChain 引入了
ParentDocumentRetriever , 所谓 Parent Document Retriever 其实就是进一步在 Document 和 Chunk 的大小之间做 trade-off,众所周知,chunk 的大小对最终交付的 RAG 效果至关重要,与其不停的在各种 size 的 chunk 大小之间上窜下跳的找最佳实践,不如路子玩儿的再野一点,在 Document
和 Chunk 之间加一层,这样一来增加了不同大小对最终效果的影响;二来在 Document 内容改变之后需要更新的时候,也可以有针对性的更新,之前我们提到可以针对 Document 做更新单位,主要是针对 chunk 做更新有些过于繁琐,但实际应用的过程中很多人反馈说针对 Document 有些过大,这下好了,中间加了一层,embedding 更新的单位也可以试着按照中间层大小,这下,大家,都满意了吧 ;)
3.
针对 RAG 系统的效果评估最近好像大家都关注的比较多,还有一个专门针对 RAG 效果评测的 Ragas(GitHub -
explodinggradients/ragas: Evaluation framework for your Retrieval Augmented
Generation (RAG) pipelines) 库,我们看到 LangSmith 也针对 Ragas 做了一些集成方面的优化,效果评估一直就是 LangSmith 的主打方向,话说回来,类似 Debug、Prompt 版本管理、效果评估、效果比较这些功能确实是当前阶段大模型开发过程里面需要的,如果有想在这一波里面通过「卖水」赚钱的同学可以好好关注一下这个方向。
思路收一下,之前的文章记录里面我们也提到过如何通过 RAY 框架优化多 PDF 文件解析、embedding
的思路,其实整个大模型应用落地的三步曲:
1.
Phase
I:RAG
2.
Phase
II:私有 FT 模型
3.
Phase
III:深度集成业务逻辑的 Agents
万事开头难,在这三个步骤里面,如何顺利推进企业内部 RAG 上线是难啃的骨头,而正确解析 PDF 等企业内部数据,为下一步 embedding 化、高效存储 emb、灵活更新 emb,又是这一堆难啃骨头里面的刺儿头,尤其是遇到文档中包含 table,甚至这些 table 还是以 image 形式存在的,控制不好不仅仅容易把 table 里面的信息打散,掐头去尾的拿去 embedding 不仅仅得不到好的结果还容易对 RAG 产生毒害。解决的方式不外乎针对 table 信息做专门的保存、解码、embedding 处理,如果是 image 那就只能上 OCR 了,信息保存部分 LangChain 前两个月发布的 MultiVectorRetriever 针对这个问题做了封装;后者类似 unstructured.io 这种封装了 tesseract 来处理(当然如果想尝鲜也可以用 Meta 8 月底新发布的 nougat 试试),上例子:
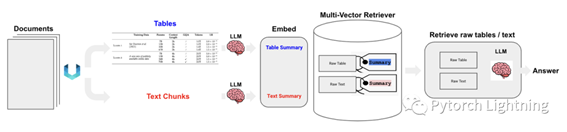
图 0:架构图
安装依赖:
o
Ubuntu 下安装
tesseract:
1 sudo apt install tesseract-ocr
2 sudo apt install libtesseract-dev
o
Ubuntu 下安装
poppler:
1 sudo apt-get install poppler-utils
o
用到的 unstructured 等包:
o
1 pip install langchain unstructured[all-docs] pydantic lxml langchainhub openai chromadb tiktoken
o
下载要解析的示例 pdf,这里用 llama2 的 papper:
o
1 # 随便加一个 useragent,否则 404
2 wget -U xxx https://arxiv.org/pdf/2307.09288.pdf
代码逻辑:
1 path = "./“
2
3 from lxml import html
4 from pydantic import BaseModel
5 from typing import Any, Optional
6 from unstructured.partition.pdf import partition_pdf
7
8 # Get elements
9 raw_pdf_elements = partition_pdf(filename=path+"2307.09288.pdf", 10 # Unstructured first finds embedded image blocks
11 extract_images_in_pdf=False, 12 # Use layout model (YOLOX) to get bounding boxes (for tables) and find titles
13 # Titles are any sub-section of the document
14 infer_table_structure=True, 15 # Post processing to aggregate text once we have the title
16 chunking_strategy="by_title",
17 # Chunking params to aggregate text blocks
18 # Attempt to create a new chunk 3800 chars
19 # Attempt to keep chunks > 2000 chars 20 max_characters=4000, 21 new_after_n_chars=3800, 22 combine_text_under_n_chars=2000)
由于涉及到 ocr,这一步时间超长,在 colab 上我花掉了 14分钟,77 页面,平均 11s/p
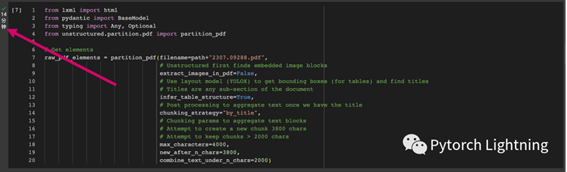
图一:OCR 部分超级耗时
大体探查一下 unstructured 解析出来的内容,可以看到有 text,有 table
1 # Create a dictionary to store counts of each type
2 category_counts = {}
3
4 for element in raw_pdf_elements:
5 category = str(type(element))
6 if category in category_counts:
7 category_counts[category] += 1
8 else:
9 category_counts[category] = 1
10
11 # Unique_categories will have unique elements
12 unique_categories = set(category_counts.keys())
13 category_count
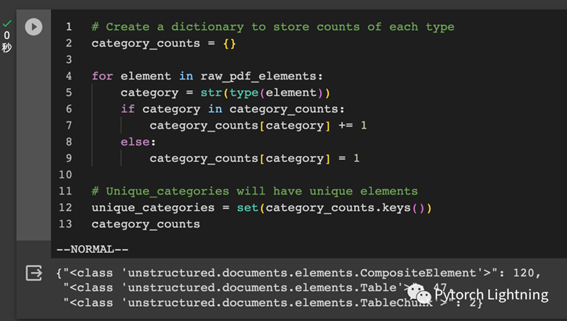
图二:unstructured 解析结果
把 text 和 table 内容分开放,以备后面继续处理:
1 class Element(BaseModel):
2 type: str
3 text: Any
4
5 # Categorize by type
6 categorized_elements = []
7 for element in raw_pdf_elements:
8 if "unstructured.documents.elements.Table" in str(type(element)): 9 categorized_elements.append(Element(type="table", text=str(element)))
10 elif "unstructured.documents.elements.CompositeElement" in
str(type(element)):
11 categorized_elements.append(Element(type="text", text=str(element)))
12
13 # Tables
14 table_elements = [e for e in categorized_elements if e.type == "table"]
15 print(len(table_elements))
16
17 # Text
18 text_elements = [e for e in categorized_elements if e.type == "text"]
19 print(len(text_elements)
20
21 ## output:
22 # 49
23 # 120
导入
LangChain 基础包:
1 from langchain.chat_models import ChatOpenAI
2 from langchain.prompts import ChatPromptTemplate
3 from langchain.schema.output_parser import StrOutputParser
4 from langchain import hub
5 obj = hub.pull("rlm/multi-vector-retriever-summarization")
这里引入了 langchain-hub 里面一个社区贡献的 prompt,看一下具体是怎么写的:

图三:multi-vector-retriever
prompt
用 LCEL 组装一个 chain,用 3.5 效果可能影响较大,能用 4 还是 4 吧。
1 # Summary chain
2 prompt = ChatPromptTemplate.from_template(obj.template)
3 model = ChatOpenAI(temperature=0,model="gpt-4")
4 summarize_chain = {"element": lambda x:x} | prompt | model | StrOutputParser()
这里可以看到通过 LCEL 这个 dsl
把各个组件串起来还是非常赏心悦目的!
组装好 summarize chain 之后就可以分别把 text 和 table 信息做提取了:
1 # Apply to texts
2 texts = [i.text for i in text_elements]
3 text_summaries = summarize_chain.batch(texts, {"max_concurrency": 5}
4
5 # Apply to tables
6 tables = [i.text for i in table_elements]
7 table_summaries = summarize_chain.batch(tables, {"max_concurrency": 5}
接下来,把 text 和 table 信息分别添加到 LangChain 的 multi-vector-retriever 里面:
1 import uuid
2 from langchain.vectorstores import Chroma
3 from langchain.storage import InMemoryStore
4 from langchain.schema.document import Document
5 from langchain.embeddings import OpenAIEmbeddings
6 from langchain.retrievers.multi_vector import MultiVectorRetriever
7
8 # The vectorstore to use to index the child chunks
9 vectorstore = Chroma(
10 collection_name="summaries",
11 embedding_function=OpenAIEmbeddings()
12 )
13
14 # The storage layer for the parent documents
15 store = InMemoryStore()
16 id_key = "doc_id"
17
18 # The retriever (empty to start)
19 retriever = MultiVectorRetriever(
20 vectorstore=vectorstore,
21 docstore=store,
22 id_key=id_key,
23 )
24
25 # Add texts
26 doc_ids = [str(uuid.uuid4()) for _ in texts]
27 summary_texts = [Document(page_content=s,metadata={id_key: doc_ids[i]}) 28for i, s in enumerate(text_summaries)]
29retriever.vectorstore.add_documents(summary_texts)retriever.docstore.mset(list(zip(doc_ids, texts)))
30
31 # Add tables
32 table_ids = [str(uuid.uuid4()) for _ in tables]
33 summary_tables = [Document(page_content=s,metadata={id_key:
table_ids[i]}) for i,
s in enumerate(table_summaries)]
34retriever.vectorstore.add_documents(summary_tables)
35 retriever.docstore.mset(list(zip(table_ids, tables)))
简单看一下 table 信息提取总结的效果:

图四:直接针对 table 做 sum 效果也是很惊艳的
组装
RAG!
1 from operator import itemgetter
2 from langchain.schema.runnable import RunnablePassthrough
3
4 # Prompt template
5 template = """Answer the question based only on the following context, which can include text and tables:
6 {context}
7 Question: {question}
8 """
9 prompt = ChatPromptTemplate.from_template(template)
10
11 # LLM
12 model = ChatOpenAI(temperature=0)
13
14 # RAG pipeline
15 chain = (
16 {"context": retriever, "question": RunnablePassthrough()}
17 | prompt
18 | model
19 | StrOutputParser()
再感叹一次,用 LCEL 来搭积木似的组装,真的很爽!
接下来测试一下 table 信息提取效果:

图五:查询表里面的信息
虽然信息的位置找对了,但看起来 ta 理解错了我的问题:
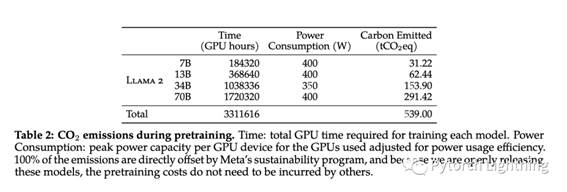
图六:原表信息
再问点稍微超纲的试试:

图七:超纲问题考验
好像说的有道理哈,总结一下:
1.
模型如果用 3.5,效果大打折扣,参见上面实验
2.
multi-vector retriever 针对 table 信息单独处理这个非常棒!
3.
ocr 对资源的消耗太大,如果是提前转换成 markdown 相信效率会高很多
出自:https://mp.weixin.qq.com/s/ff9fn0FDlpDrEePzJFOEQQ
本文档由网友提供,仅限参考学习,如有不妥或产生版权问题,请联系我们及时删除。
客服请加微信:skillupvip